A multivariable control matrix has manipulated variables (MVs) on one axis, controlled variables (CVs) on the other axis, and models in the matrix that indicate a relationship between that MV/CV pair. Effective multivariable controllers use the right models for various control and optimization needs.
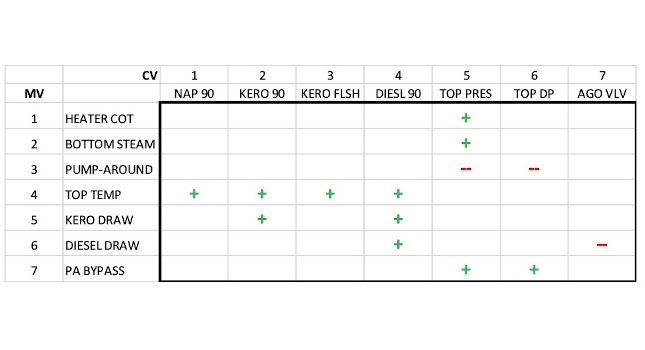
Matrix design practice refers to how control engineers go about designing the matrix at the heart of any multivariable controller. A multivariable control matrix consists of manipulated variables (MVs) along one axis, controlled variables (CVs) along the other axis, and models at various locations within the matrix, which indicate a relationship between that MV/CV pair (Figure 1). Designing the matrix consists of selecting the MVs, CVs and models which, for various control and optimization purposes, are wanted in the multivariable controller.
“Large-matrix” design practice has been dominant (nearly exclusive) in industrial applications for the last 30 years. In large-matrix practice, a wide-ranging plant test is used to identify all related process variables and process interactions (models). An underlying principle has been more variables and more models yield a more complete solution, for both control and for optimization purposes. It has been routine for large-matrix practice to result in one large multivariable controller spanning an entire plant unit, with dozens of variables (sometimes more than 100) and hundreds of models (sometimes upwards of 1,000).
A side-effect of this approach is most installed multivariable controllers in the process industry today are large, complex, fragile, costly, and challenging to own, operate and maintain. Moreover, the large-matrix approach has excluded smaller multivariable control applications that may be warranted based on more basic control and operation improvements, but which do not necessarily justify the high cost threshold of the large-matrix paradigm.
In conventional multivariable control technology, the built-in optimizer always has been considered an essential piece, so most involved never questioned it. Instead, the search has looked for better ways to support and maintain the large-matrix controllers. However, with insight from decades of experience, it is now possible to see alternative ways to build smaller and more efficient multivariable control applications to address operation and control needs without a built-in optimizer, and therefore without the side-effect of growing the matrix beyond its basic control and operation scope.
Operation-driven matrix design
Operation-driven matrix design can reveal important automation opportunities that have previously remained “below the radar” of large-matrix practices. (See “Low altitude radar: Smaller multivariable control.”) It differs from optimization-driven design in key ways. It often results in smaller multivariable controllers, rather than one large controller, with fewer variables and models. When concerns arise regarding a variable’s importance, its efficacy for control, or the reliability of its models, it is often left out of the matrix. Leaving it out errs on the side of reliability. Keeping it errs on the side of optimization. The overarching goal of operation-driven matrix design is automation, not optimization, and such a design strives to include only necessary, reliable, and worthwhile parts.
In operation-driven matrix design, groups of related single-loop controllers are identified, based on frequent (often highly coincident) changes to setpoints, outputs or modes. This activity represents manual open-loop multivariable control being carried out by the operating team. The objectives are to:
- Automate these manual multivariable control scenarios to capture the well-known intrinsic benefits of closed-loop vs. open-loop control
- Relieve the operating team of repetitive control loop micro-management, thereby allowing more time to play a proactive process oversight role.
Operation-driven design does not strive to include all related variables and models. It often focuses on the ones already used in existing manual multivariable control operating practices and procedures. It automates established proven procedures and captures the experience and skill of the operating team regarding which MVs are suitable and reliable for controlling which CVs. Using automation to capture operator skills is an important criteria that large-matrix practice often neglects in favor of greater optimization.
Where does this leave optimization?
Optimization belongs in the business layer, based on access to site-wide information, ability to bring a variety of planning, scheduling, blending and modeling tools to bear, and the appropriate execution time frame, which is typically daily and not in real time. Common practice is performing daily updates to the (optimized) site-wide production plan and handing it down via the operating chain of command, usually starting with the “morning meeting.”
This raises questions about the conventional multivariable control built-in optimizer in the control layer. In the control layer, multivariable control needs to execute at high frequency because process values are subject to real-time changes. However, optimization normally does not need to execute at high frequency because optimization normally does not change in real time. This makes the control layer optimizer redundant and unnecessary, even as it contributes significantly to the high cost and maintenance of conventional multivariable control applications (second only to model maintenance).
Low altitude radar: Smaller multivariable control
Many smaller multivariable control applications have remained “below the radar” of the large-matrix multivariable control paradigm, but the exact nature of these applications and a method to identify them has never been proposed — until now. A simple technique for spotting these applications is to use a console activity log to reveal the controllers that require the most operator intervention in the form of setpoint, output or mode changes (Figure 2).
A safe assumption is most of these “bad actors” are effectively in a manual multivariable control scenario, wherein operators must frequently adjust groups of related controllers to keep related process variables within constraint limits and (to the extent possible) at optimum target values. [Note: the term “optimum target values” refers to how remaining MV availability (after constraint control) is utilized, and does not necessarily mean the value comes from a real-time optimizer, which in fact it often does not.]
This manual open-loop activity can be automated — these multivariable loops can be closed — by deploying an operation-driven multivariable controller to capture closed-loop control benefits vs. open-loop control.
This same technique has successfully been used in industrial applications twice in recent memory: Once to address loops-in-manual (as opposed to multivariable loops in manual) and once to address “bad actor” alarms. In each case, this technique was used to show the extent of the problem, and then measure progress towards best practice target metric values. According to EEMUA 191, Alarm systems – a guide to design, management and procurement, operators should not have more than one alarm per 10 minutes. However, there’s no best practice guideline for controller interventions per hour. This also has remained below the radar — until now.
Allan Kern, P.E., is owner, president, and control engineering consultant at APC Performance LLC. Edited by Mark T. Hoske, content manager, Control Engineering, CFE Media, [email protected].
MORE ANSWERS
KEYWORDS: Advanced control, multivariable control
Multivariable control can pursue optimization targets without having its own optimizer. This is already (has long been) the norm throughout process control.
By focusing on control and operation needs, rather than optimization, matrix design can be streamlined, leading to more efficient and reliable multivariable controllers.
All optimization will likely reside in the business layer (as most always does), rather than in the control layer, where it is redundant and problematic.
CONSIDER THIS
Are you updating your multivariable control implementations to close more loops?