Explore essential factors to optimize the performance and reliability of data acquisition (DAQ) systems in industrial settings.
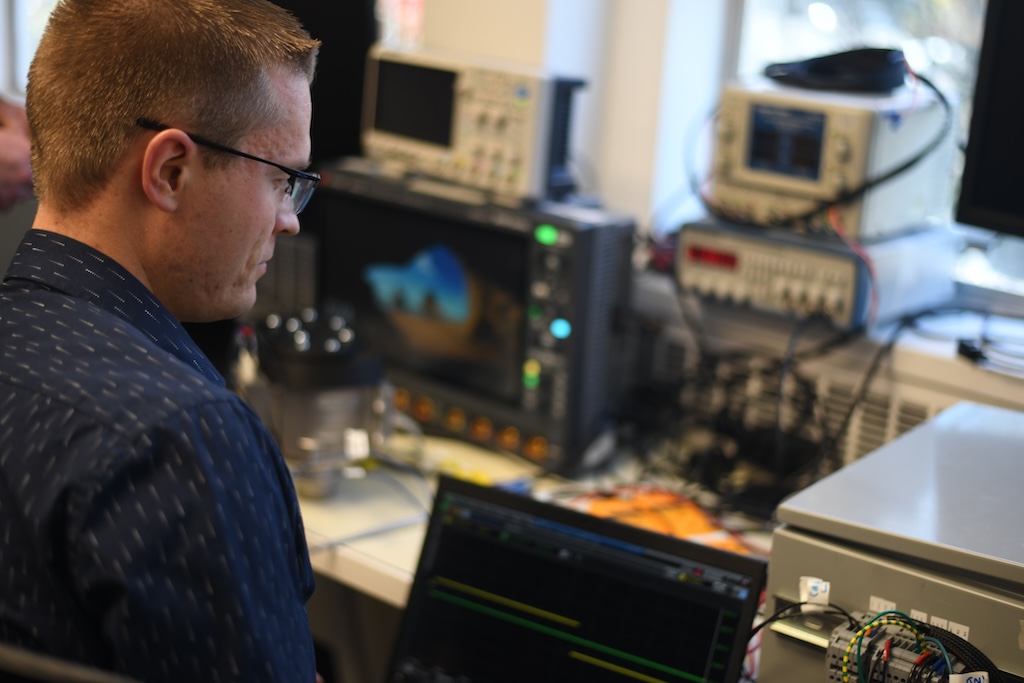
Learning Objectives
- Learn strategies to minimize data collection while meeting system demands for optimized performance.
- Differentiate between differential and single-ended sensor inputs and their implications on noise reduction and system performance.
- Understand the importance of strategic data management in preventing issues related to long-term storage and resource utilization.
Data acquisition insights
- Data acquisition (DAQ) systems in industrial sectors are crucial for monitoring and controlling processes, ensuring quality, efficiency, and safety across various industries.
- Effective DAQ system design requires careful consideration of measurement demands, appropriate sampling rates, and proper signal conditioning to ensure accurate and meaningful data collection.
- Optimizing data integrity involves selecting the right sensors, managing noise through filtering, and periodically verifying the data to ensure it aligns with expectations and avoids unnecessary complexity.
Data acquisition (DAQ) in the industrial sector plays a critical role in monitoring and controlling processes. These are essential systems for ensuring quality, efficiency and safety for a variety of industries. However, to optimize their performance and reliability, there are several considerations to be aware of. This article explores key factors to consider in the design and implementation of DAQ systems in industrial applications.
A common misconception in practice is that simply collecting data and maximizing available information is the best strategy, when careful consideration would yield better outcomes. Not only should the system be implemented with careful consideration, but the data that it produces should be verified after a few days, and periodically after a few months. This ensures that the collected data is truly useful and aligns with expectations.
Consider this scenario: a data acquisition system continually logging zeros for months or even years because no one reviewed the data or cross-checked the numbers with historical trends. Unfortunately, this situation occurs far too frequently. The following three parts will discuss how to avoid this pitfall.
Foundations of effective DAQ systems
The foundation of any DAQ system lies in its ability to meet specific measurement demands. One must carefully consider what the data is for and how it will be applied. The strategy should focus on obtaining as little data as possible to meet the demands of the system and for retention. Consider determining which physical parameters need to be measured (such as temperature, pressure or flow rate), the range of these measurements and the required level of accuracy. The selected DAQ system must possess the capability to accurately capture the required data across all anticipated operating conditions.
Once the initial requirements are understood, diving into the details becomes an important next step. Selecting the appropriate sampling rate is vital for capturing accurate data. The sampling rate should be high enough to accurately represent the signal being measured, but not so much that it loses meaning in the data.
According to the Nyquist Theorem, the sampling rate should be at least twice the highest frequency present in the signal to avoid aliasing. However, excessively high sampling rates can lead to large data volumes, increasing storage and processing requirements. This should be avoided. Take, for example, temperature monitoring. Typically, thermal processes have a time constant of minutes, so sampling at hundreds of kHz is meaningless and filled with noise. However, sampling and averaging before logging can be effective to avoid the need for additional hardware filters or sampling at a slower speed, both of which work.
Once the sampling rate is determined, signal conditioning becomes a pivotal aspect of DAQ systems, involving the manipulation of an acquired signal to make it suitable for processing. This ties back into the question of band limiting to avoid noise and excessive data collection. Filtering can ensure that your data has a lower noise platform and provides more meaningful information. Proper signal conditioning ensures the integrity of the signal and prevents corruption, thereby enhancing the overall quality of the acquired data.
Optimizing data integrity
Always design and check to ensure proper data quality. There are three areas of consideration: the first is the analog sensor’s operating range, the second is the analog-to-digital conversion bit resolution and the third is noise management through filtering or common mode subtraction. The sensor needs to be selected so that the operating region covers nearly the full range of the sensor. So, if measuring 100 PSI of pressure as typical, choose a sensor that covers that range plus the typical maximum and minimum. For the analog-to-digital conversion, ensure there is the bit resolution to capture the data precision needed. Analog-to-digital conversion is typically 8, 12, 16 or 24 bits. Higher bit ratings are more expensive but can acquire higher data quality.
Choosing the appropriate range typically boils down to selecting the right sensor. The sensor is just as crucial as any other component in the DAQ system. It should not only meet the input requirements under normal conditions but also withstand extreme conditions. Take a moment to review various scenarios to ensure that the right part is chosen. I have frequently observed this overlooked, especially with pressure sensors and accelerometers, where the input and shock are not consistently linear. Consider extreme cases where data may not need to be captured but acknowledge that sensor saturation is acceptable during those events.
Reducing noise in a signal often involves choosing between differential and single-ended sensor types. Differential inputs — two high impedance inputs that subtract between the two — are preferable for long cable runs or in environments with significant electrical noise, as they offer significantly better common carrier noise rejection. Conversely, single-ended inputs are suitable for shorter runs and low-noise environments, providing a simpler and often more cost-effective setup. Many 4-20 mA sensor applications employ the two-wire system, which is a single-ended type system. Despite its appearance as differential, the input impedance differs on each wire. The decision between these two should consider both the operational environment and cost implications.
Finally, once the data is being logged, take a moment to check the numbers and verify that they have meaning. This should be done in two ways. The first is to look at the individual numbers and verify that they are truly measuring what is happening. The next and often omitted, step is to return a few hours to days later and create a graph of the data. Look at it and verify that it makes sense. It is surprising to find out how often the graphed data or the act of creating the graph reveals the data’s limitations. Make the necessary corrections to your system at that time.
Strategic data management and analysis
In data acquisition, understanding the exact data required is paramount. Acquiring only the minimum necessary data can prevent issues linked to long-term storage and buffering, which might compromise the system. It is important to resist the temptation to over-collect data in replacement of considering its necessity. Without a proper analysis of needs, excessive data collection can result in needless complexity and unmanageable resource usage.
Regular checks for errors, such as gaps or noise in the data, are crucial. One effective strategy is to acquire high-resolution data in the short-term and then aggregate it for long-term logging. This approach ensures detailed data availability when needed, while also managing data volumes over time. For instance, in power data analysis, maintaining high resolution for the first week is essential, followed by lower resolution for monthly data and aggregated yearly data by month. Valuing data appropriately is key.
With data collection “less is more”
Optimizing data acquisition in industrial settings demands a thorough understanding of measurement requirements, careful sample rate selection, appropriate signal conditioning, alignment of resolution with sensor input range, prudent sensor selection and a strategic choice between differential and single-ended inputs. By addressing these factors, industries can ensure the capture of top-tier data, essential for process optimization, efficiency enhancement, and safety assurance. Moreover, following the “less is more” principle and proficiently managing long-term logging can foster a more efficient and dependable DAQ system.
Dr. Michael Wrinch is the founder of Hedgehog Technologies. Edited by Tyler Wall, associate editor, Control Engineering, WTWH Media, [email protected].
KEYWORDS: Data acquisition systems, data management, optimizing data
CONSIDER THIS
How can data acquisition enhance your manufacturing operations?