There are both opportunities and complexities involved when implementing artificial intelligence (AI) at the industrial edge, highlighting the role of specific hardware, example use cases, regulatory impacts and strategic considerations for sustainable, adaptable AI investments.
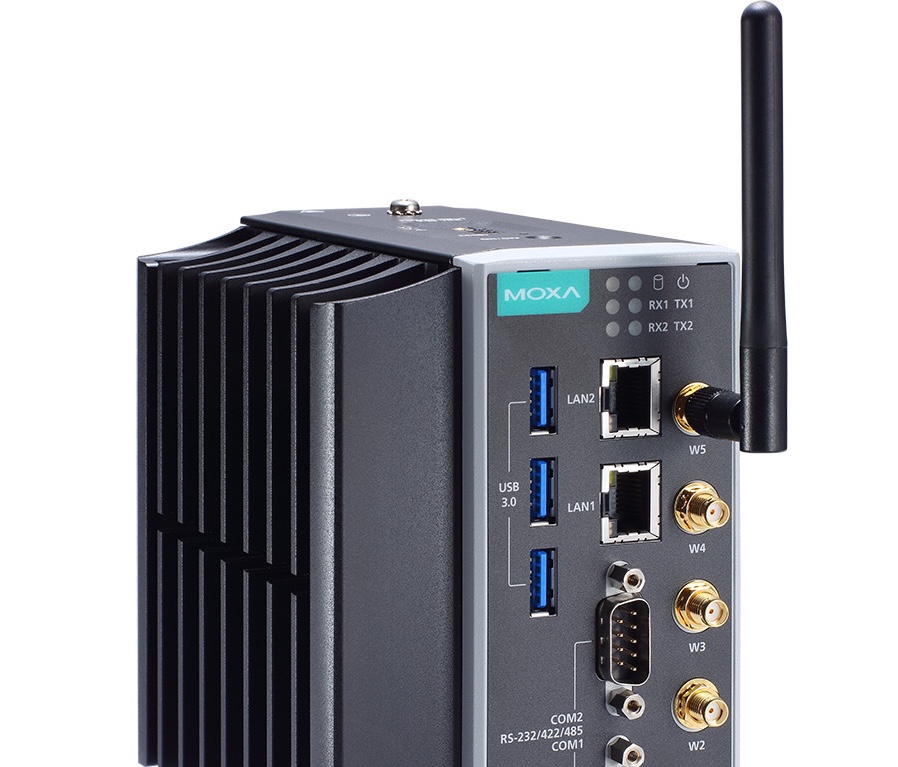
Industrial edge insights
- Artificial intelligence (AI) is becoming increasingly accessible with rapid growth and change seen across various software and hardware platforms.
- It may be easier for predictive maintenance applications to leverage AI using incremental investments and existing architectures.
- Regulations may create barriers or shift the economics of AI solutions, including how AI tools can be applied, whether by imposing additional restrictions, mandating specificÂ
Much like the Internet of Things (IoT) and cloud computing before it, artificial intelligence (AI) has all the hallmarks of being a transformative technology with tremendous potential to enhance industrial operations. As AI becomes increasingly accessible, rapid growth and change is already being seen across various software and hardware platforms, as well as among a wide array of providers. We can also expect high volatility in demand, economic conditions, available licensing and commercial models and the regulatory landscape.
For technology leaders, engineers and decision-makers, this presents both significant challenges and promising opportunities. Navigating these complexities will be essential to making informed investments in the enabling technologies that can unlock AI’s full potential at the industrial edge.
Enabling AI for local edge computing
The practical application of AI at the edge will rely on both the effectiveness of the inference model and where that inference model needs to be located to be effective in each use case. In many cases, the use case requires a local instance of an inference model on an edge computing device that can respond in real-time instead of sending requests to a cloud or central server. The choice of hardware will fall into one of these options based on the specifics of a given application and inference model:
- Graphics processing units (GPUs): Known for parallel processing, GPUs excel in handling large AI workloads like training complex neural networks. They are highly efficient for tasks requiring significant matrix computations, such as image and video processing. However, GPUs can be power-hungry and costly for edge deployments.
- Neural processing units (NPUs): Designed specifically for AI tasks, NPUs provide excellent performance for inference at the edge, offering high efficiency in low-power, embedded environments. While great for specific AI tasks, NPUs are often less flexible and not suitable for general-purpose processing.
- Central processing units (CPUs): Versatile and widely used, CPUs handle various tasks, including simpler AI computations and are beneficial for applications that don’t require heavy processing. While CPUs historically lack the parallelization and efficiency needed for high-intensity AI workloads, CPU manufacturers are already responding by introducing new options with different levels of NPU and GPU integration.
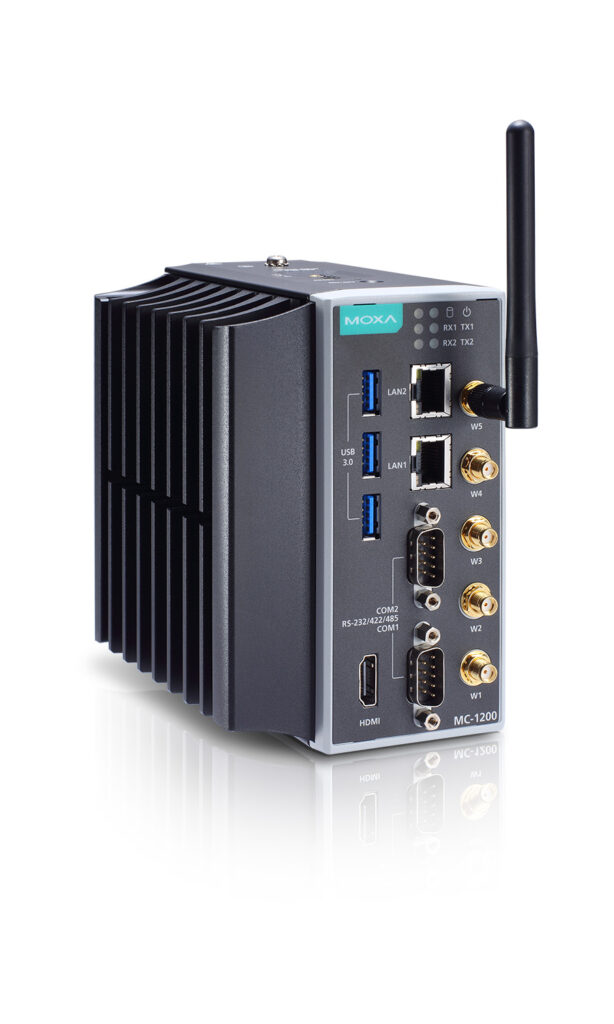
Using AI for video-based leak detection
A promising use case for AI at the industrial edge is for leak detection. With an inference model that can quickly identify potential gas leaks from live video footage, organizations have the potential of achieving continuous and cost-effective real-time leak detection across hundreds of sites.
However, if that model relies on a powerful computer and GPU, it may be difficult to find suitable hardware to run that model reliably in remote and harsh environments. It may also be extremely challenging to rely on a centrally located server to host the inference model if the existing network architecture isn’t able to handle the bandwidth or latency requirements.
Specifics about the inference model can also have large implications in terms of the type of investment and engineering effort required to deploy an AI-powered leak detection solution at scale. For example, with an inference model developed in-house using Nvidia GPUs, the choice may be between investing in the enclosure and cooling apparatus for the necessary computer hardware or waiting for manufacturers to offer more capable, rugged compute models. With an inference model that is platform-independent, other options may become available, such as using a power-efficient NPU or AI accelerator that optimizes performance for specific AI computational tasks.
These architectural considerations are also seen with other use cases that involve live analysis of massive streams of data, such as the 3D point clouds generated by LiDAR sensors. Additional considerations specific to each use case may also emerge, such as the effectiveness and maturity of inference models.
Leveraging AI for predictive maintenance
In contrast to computationally intensive real-time applications like video analysis, it may be easier for predictive maintenance applications to leverage AI using incremental investments and existing architectures. Critically, systems may already be in place to collect valuable data through programmable logic controllers (PLCs) and industrial IoT platforms, and these systems can be leveraged to support a predictive maintenance platform using AI. Since predictive maintenance does not have the same reliance on immediate real-time analysis of massive amounts of data, the inference model can reside on a central or cloud server, and the edge device is simply responsible for easy, cost-effective equipment data collection.
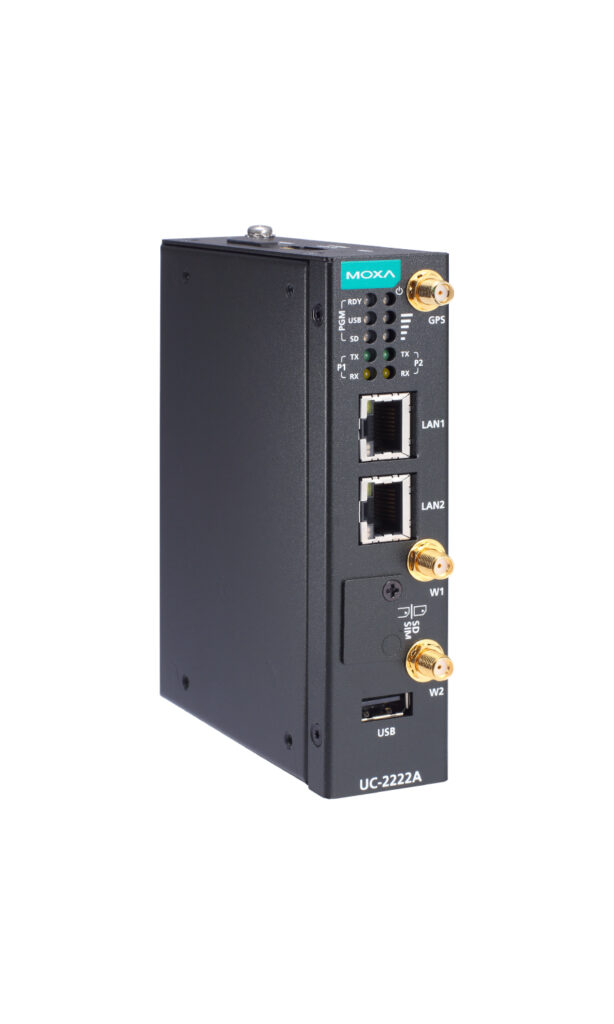
With predictive maintenance, it may also be feasible for the edge device to run inference models locally, without need for special hardware like a GPU or NPU. Highly optimized predictive models can operate on light, low-power processors, such as Arm-based systems. This is also a natural progression for many customers that found initial value using Industrial IoT to gain real time visibility into their devices and processes and now see a value in leveraging that data for more efficient operation and maintenance.
Regulatory and other considerations for edge computing
The regulatory landscape surrounding AI introduces both challenges and growth opportunities for industrial applications at the edge. For instance, regulations on emissions reporting could drive demand for AI-based leak detection solutions that leverage edge processing, allowing for comprehensive monitoring at a fraction of the cost of traditional systems. Similarly, regulations on managing or reducing energy use may open doors for AI applications that can swiftly pinpoint areas of energy waste and optimize operations.
However, regulations can also create barriers or shift the economics of AI solutions. For example, if new classifications for certain technologies emerge, this could alter how AI tools can be applied, whether by imposing additional restrictions, mandating specific compliance measures or altering incentives for imports and exports of AI technologies.
The rapidly evolving AI landscape also brings the risk of market disruptions, as new players emerge with innovative solutions, incumbents pivot or mergers and acquisitions alter the available product offerings and the economics of support. For example, the introduction and effectiveness of ChatGPT’s large language model has completely altered the landscape and perception of how AI can be made practical and caused other companies to quickly pivot in response or even build new commercial offerings based on running queries to the GPT engine It remains to be seen how new AI platforms from Apple, xAI, Meta, Anthropic and others will create new opportunities or render an existing solution obsolete.
Organizations need to be vigilant about these market shifts and regulatory changes to protect their investment and ensure long term success and competitiveness. Given the high volatility that can only be expected in such an exciting and fast-growing market, it is also important to consider the risk inherent in having your solution dependent on ready availability to these platforms, especially with large unknowns like future licensing fees or legal concerns over ownership of training data and queries.
AI is a powerful tool for edge computing
AI at the industrial edge is quickly becoming a powerful tool, bringing potential for transformative efficiency, predictive insights and operational resilience. Yet, it also requires decision-makers to carefully consider hardware trade-offs, evolving use cases, regulatory implications and the flexibility needed to adapt as new technologies and players emerge. As with any technology at the forefront of innovation, the journey to fully realizing AI’s potential in industrial settings will be marked by navigating a dynamic landscape. Thoughtful, strategic investments today can lay the foundation for sustainable growth and a competitive edge in the future.
Oliver Wang is the Product Marketing Manager for Moxa Americas’ industrial computing lines. Edited by Sheri Kasprzak, managing editor, Automation & Controls, WTWH Media, [email protected].