Edge AI provides many benefits for manufacturers including reduced latency, better reliability, cost reduction and more.
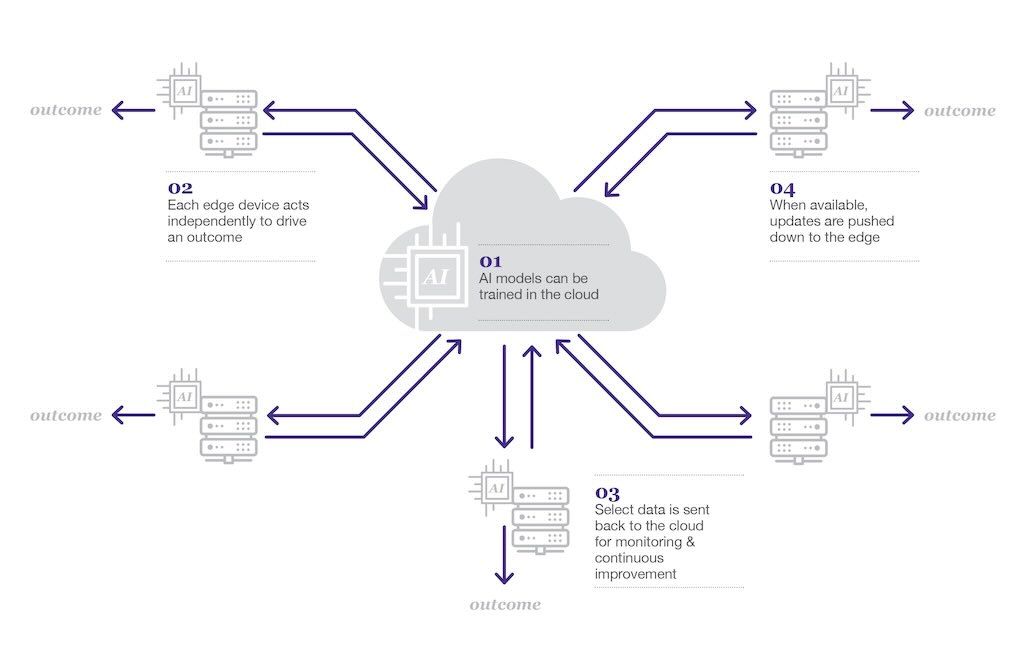
Learning Objectives
- Understand the advantages of edge AI over cloud AI, including reduced latency, enhanced security and increased flexibility for diverse industry applications.
- Explore how edge and cloud AI complement each other and recognize key industry players collaborating to merge these technologies for more robust AI systems.
- Identify the critical aspects of an edge infrastructure that empower AI transformation, enabling organizations to drive competitive advantages in the evolving AI landscape.
Edge AI insights
- AI’s evolution from theory to practice is evident as organizations prioritize AI projects, highlighting its transformative potential and increased accessibility.
- Edge AI, migrating from the cloud, offers benefits like reduced latency, enhanced security and cost efficiency, shaping the future of AI-driven solutions.
The artificial intelligence (AI) landscape has undergone a rapid transformation from a realm of theoretical possibilities to tangible applications reshaping industries worldwide. Today, almost three-quarters of organizations are prioritizing AI projects over other digital initiatives, which underscores the increased accessibility and AI’s potential transformative impact.
While AI technologies have often found their home in the cloud, AI solutions are migrating to the edge as the technology matures. Termed “edge AI,” these applications are run on or near the end device(s). They reduce latency, improve security, provide privacy, enhance flexibility and more. The burgeoning edge AI market is projected to grow at a robust compound annual growth rate (CAGR) of 20.8% and reach $39.61 billion by 2032.
Five edge AI benefits
The growth of edge AI underscores the benefits edge computing provides to full-scale AI deployment.
-
Reduced latency: Many emerging AI applications, like collaborative automation and defect detection, require near-instantaneous processing. Edge AI is able to meet these requirements by processing data at the edge, thereby eliminating the need for data to be transmitted and processed at a central cloud server.
-
Reliability and resiliency: Edge AI also is able to provide better reliability and resiliency by processing data at the edge. Because the technology does not rely on network connections, even if the network is compromised, the AI can function as a standalone thereby minimizing the impact to the organization. This is important in critical applications such as public safety and healthcare.
-
Cost reduction: As AI workloads grow, scaling up resources on a cloud-only architecture can become too expensive due to increased bandwidth usage and cloud storage costs. Further, there are often limits to how much a cloud provider can scale their GPU resources. By leveraging edge AI, companies can have more flexibility in how they scale their solutions while curtailing expenses by leveraging local processing.
-
Avoiding vendor lock-in: Relying only on a single cloud provider’s AI service can lead to vendor lock-in, making it difficult and expensive to switch providers due to the resources needed to transfer data and models as well as the egress fees incurred.
-
Meeting security, privacy and compliance needs: Sending sensitive data like customer information or proprietary designs to a public cloud for training AI models raises security and privacy risks. This is especially true in certain industries such as healthcare and finance where strict data regulations make public AI cloud services difficult to use. Leveraging on-prem computing, especially when it can meet cybersecurity certification requirements, can be one way to satisfy security needs.
The complimentary relationship of the edge and cloud
While edge AI presents significant advantages, it is not the sole solution. Ai’s future lies in the edge and cloud environments. By providing consolidated data across multiple sources, space for open-source collaboration, and new development tools that improve accessibility, the cloud is second-to-none in its ability to foster the development and enhancement of AI solutions.
For most applications, though, the edge wins out once the solution is ready to scale. It provides the speed, flexibility, reliability and cost to enable success in the field. Taken a step further, select data sets can be sent from edge devices to the cloud where the solution can be further refined with updates being pushed back down to edge devices. In this way, companies can leverage the best of both environments to create an adaptable, future-proof solution.
The edge AI ecosystem
Where AI was once ubiquitous with cloud computing, several companies are now leading the charge to the edge. Hardware players are providing more technologies to enable powerful computing in smaller, more efficient devices.
Cloud companies also are enabling edge technologies to complement their cloud services. These companies, through new products and partnerships, are expanding the infrastructure to include edge services, helping ensure a seamless experience across cloud and edge environments.
GPU innovators and cloud providers are marrying their technologies with those of edge industry leaders like Eurotech who have made their business developing the framework that has enabled digital transformation through the capture, communication, and computation of data from field devices. With deep industry experience, these partners build the infrastructure required to fuel and deploy AI models at scale while also paving the way for an interoperable edge that will allow seamless integration of AI solutions across various devices and platforms.
Three steps to building an edge AI infrastructure
The importance of developing this digital infrastructure cannot be understated. Despite the emphasis companies are placing on AI adoption, only 54% of AI initiatives move past the proof-of-concept stage. These projects often stall as companies lack the data to develop an AI model that drives meaningful business outcomes. In a case of “measure twice, cut once,” companies can help ensure the success of their AI initiatives by developing a digital infrastructure, dubbed the artificial intelligence of things (AIoT) that’s able to provide quality data to develop impactful AI models, thereby setting the stage for immediate AI adoption and future AI growth.
1. Data capture: The AIoT must incorporate data from all pertinent sources. This often means collecting data from a variety of equipment including legacy OT systems and sensors. Working with organizations with experience in developing IoT solutions in a specific industry can help mitigate the challenges this process presents. These companies can leverage protocol libraries and purpose-built edge hardware to collect and clean edge data.
2. Data synchronization: Data collection is only one piece of the puzzle, however. Data collected from any given source is monolithic until it is synchronized with the data from other sources. Data synchronization is the process of establishing consistency within data sets so they can be used by machine learning (ML) models.
Developers must account for a litany of potential challenges like network inconsistencies, data changes, asynchronous syncs, server errors and more. Experienced IoT partners can help solve this issue.
One option is leveraging a digital twin. Once created, the digital twin acts as a sole source of truth where incoming data can be compared and synchronized. As a result, the digital twin becomes the real-time, accurate representation of the physical asset or system from which the AI can be powered.
3. System security: The development of this AIoT infrastructure opens operational technology (OT) systems to new, significant risks. In fact, according to a report by Fortinet, 61% of cybersecurity intrusions affected OT systems in 2022. Bringing computing to the edge is one significant step in reducing security concerns. It reduces the amount of communication outside the contained system, thereby reducing the “openings” bad actors can exploit.
Companies can further mitigate their risk by building safeguards throughout their solution — from physical hardening of edge devices to a secure “chain of trust” for data communication.
The good is news there are standards that outline cybersecurity best practices. Foremost among these is ISA/IEC62443, which covers every stage and aspect of industrial cybersecurity from risk assessment to operations. Companies should seek out certified solutions to meet this or equivalent cybersecurity standards from the edge to the cloud.
The evolution of ML from theoretical concepts to practical AI applications has ushered in a new era of innovation and efficiency across industries. While AI has historically been a primarily cloud technology, new AI capabilities are driving AI to the edge where users benefit from reduced latency, enhanced security and increased flexibility.
As organizations embrace edge AI solutions, they are poised to unlock unprecedented opportunities for real-time decision-making and improved operational effectiveness.
The projected growth of the edge AI market underscores the growing importance and potential impact of this technology in shaping the future of AI-driven solutions. By leveraging the strengths of edge and cloud AI, businesses can create more robust and adaptive AI systems that cater to diverse needs while driving sustainable growth and competitive advantage in the dynamic landscape of AI.
Lindsay Hilbelink is global strategic marketing manager for Eurotech. Edited by Chris Vavra, senior editor, Control Engineering, WTWH Media LLC, [email protected].
Eurotech is a partner member of the Control Systems Integrators Association (CSIA).
Keywords: edge computing, edge AI
CONSIDER THIS
What are your considerations for choosing edge AI over cloud AI?
REFERENCES
Edge AI Market Size, Share, Trends, Growth & Forecast | 2032 (futuremarketinsights.com) (or can cite CFE who also used this stat: Edge AI market projecting strong growth (controleng.com))
Gartner Survey Reveals 80% of Executives Think Automation Can Be Applied to Any Business Decision